VertNet: Accurate Vertebra Localization and Identification Network from CT Images |
Zhiming Cui1,2,5, Changjian Li3, Lei Yang2, Chunfeng Lian4, Feng Shi5 |
Wenping Wang2, Dijia Wu2, Dinggang Shen2 |
1ShanghaiTech University, 2The University of HongKong, 3University College London |
4Xi’an Jiaotong University, 5Shanghai United Imaging Intelligence Co. Ltd. |
International Conference on Medical Image Computing and Computer Assisted Intervention (MICCAI), 2021 |
|
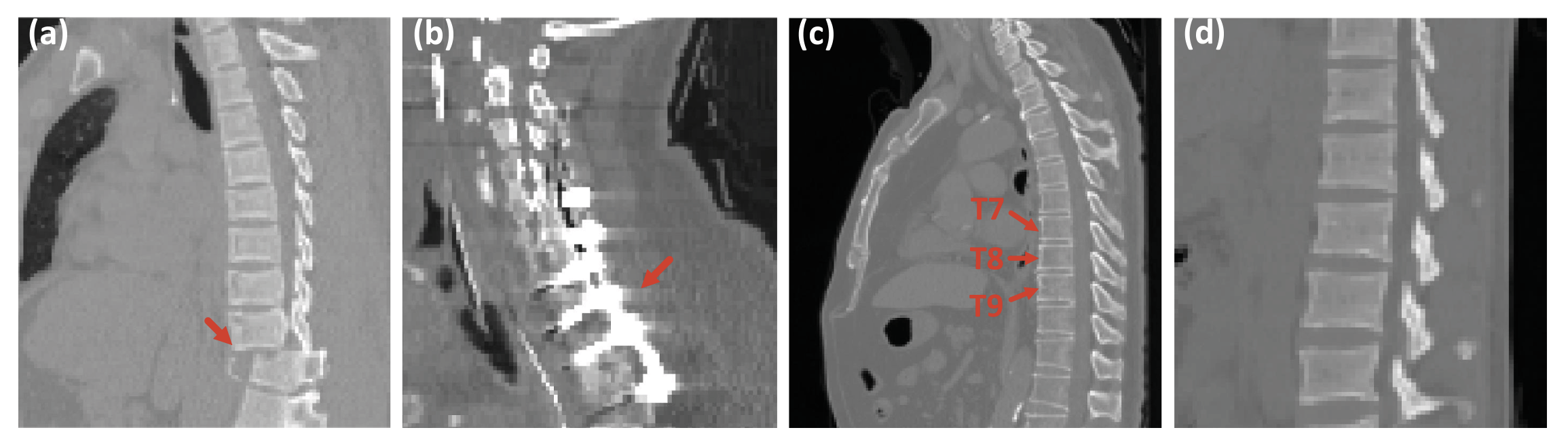 |
Fig. 1. Typical challenging cases: (a) Spine with pathological fracture; (b) Image with metal artifacts; (c) Adjacent vertebrae having similar shape appearance; (d) Image with a limited field of view. |
Abstract |
Accurate localization and identification of vertebrae from CT images is a fundamental step in clinical spine diagnosis and treatment.
Previous methods have made various attempts in this task;
however, they fail to robustly localize the vertebrae with challenging appearance or identify vertebra labels from CT images with a limited field of view.
In this paper, we propose a novel two-stage framework, VertNet, for accurate and robust vertebra localization and identification from CT images.
Our method first detects all vertebra centers by a weighted voting-based localization network. Then, an identification network is designed to identify the label of each detected vertebra in leveraging the synergy of global and local information. Specifically, a bidirectional relation module is designed to learn the global correlation among vertebrae along the upward and downward directions, and a continuous label map with dense annotation is employed to enhance the feature learning in local vertebra patches.
Extensive experiments on a large dataset collected from real-world clinics show that our framework can accurately localize and identify vertebrae in various challenging cases and outperforms the state-of-the-art methods.
|
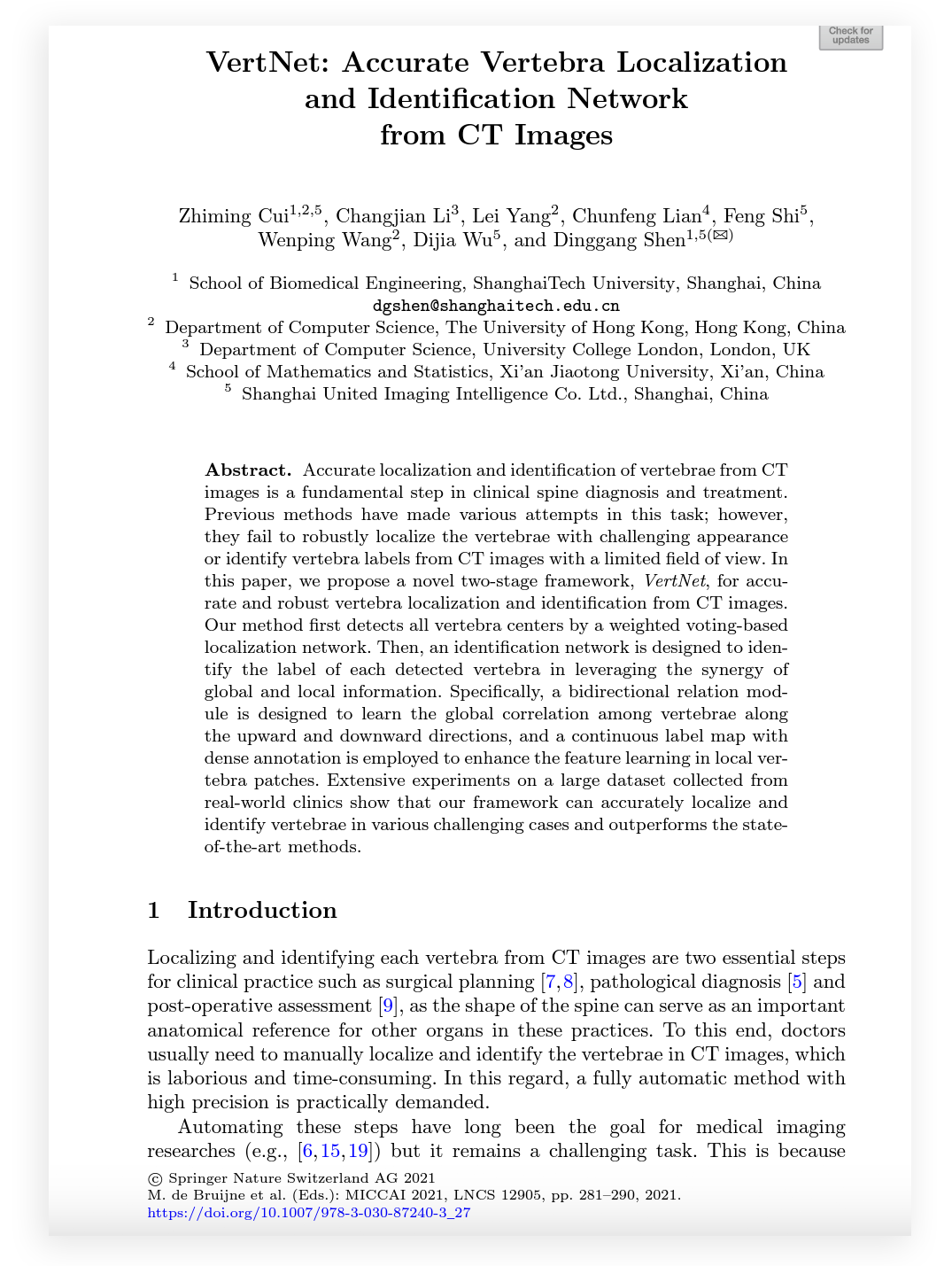 |
|
Paper [PDF]
Data [Code and Data (coming soon...)]
Citation: Cui, Zhiming, Changjian Li, Lei Yang, Chunfeng Lian, Feng Shi, Wenping Wang, Dijia Wu, and Dinggang Shen. "VertNet: Accurate Vertebra Localization and Identification Network from CT Images." In Medical Image Computing and Computer Assisted Intervention–MICCAI 2021: 24th International Conference, Strasbourg, France, September 27–October 1, 2021, Proceedings, Part V 24, pp. 281-290. Springer International Publishing, 2021. (bibtex)
|
|
|
Algorithm pipeline |
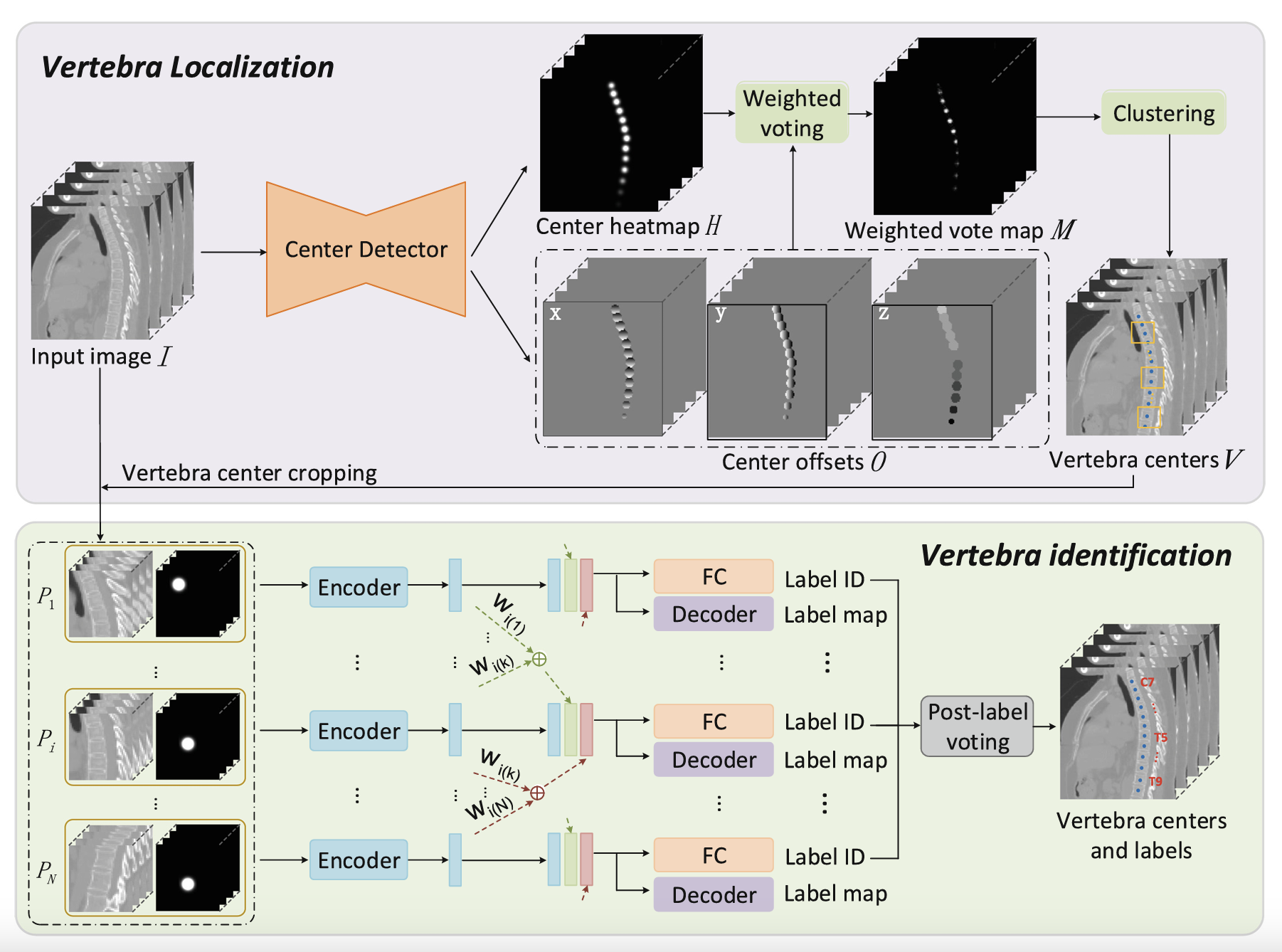 |
Fig. 2. An overview of the proposed VertNet for vertebra localization (Sec. 2.1) and identification (Sec. 2.2) from input CT images.
|
|
Representative Results |
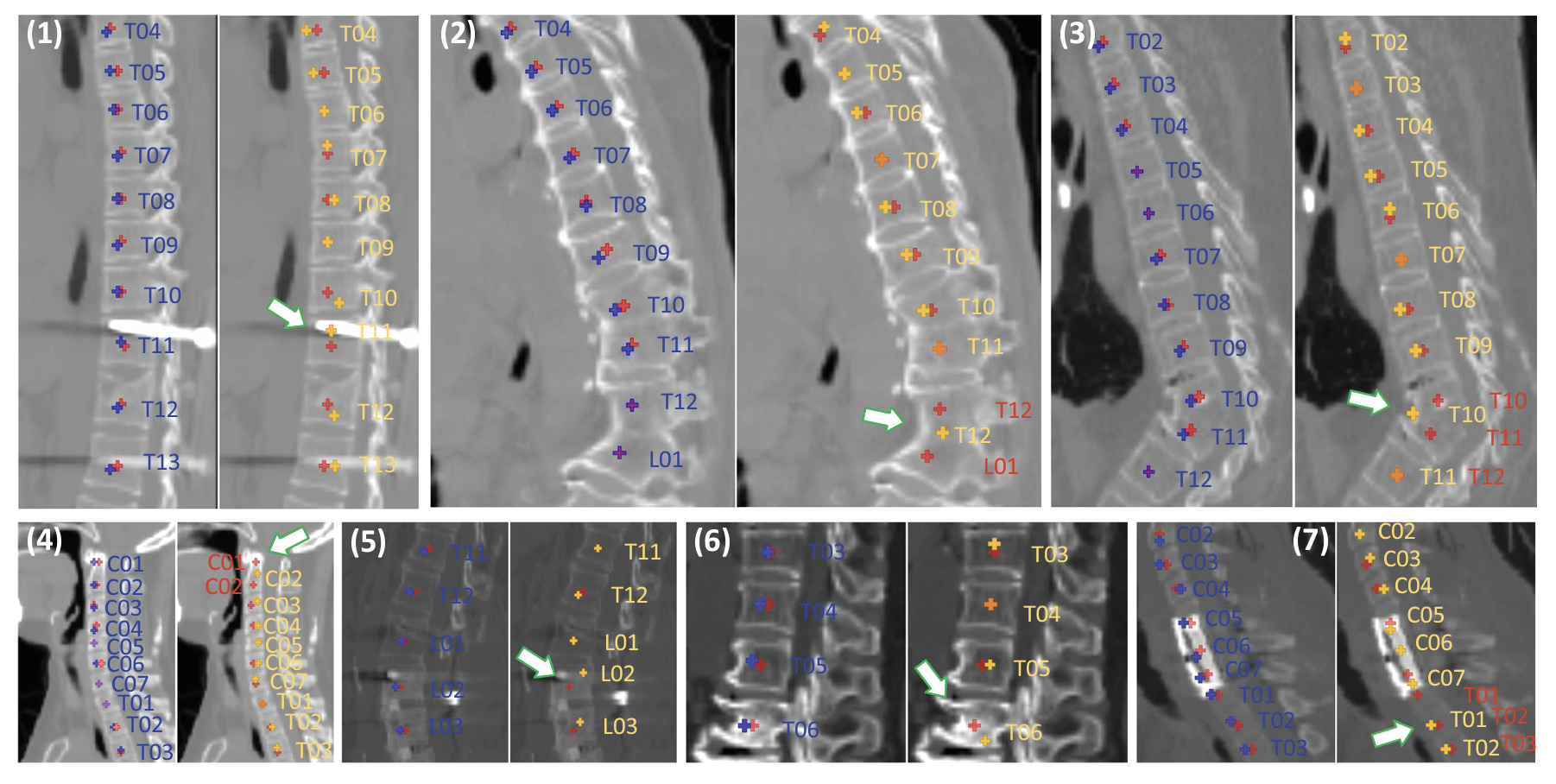 |
Fig. 3. Comparison between our results (blue) and those by Deep-HMM (yellow) against the ground-truth (GT) (red). Seven typical examples are presented: metal artifacts(1,7), pathological spines(2,3), and limited field of view (4,5,6,7). The GT label is annotated if incorrect prediction occurs. (Color figure online) |
|
|
©Changjian Li. Last update: October 21, 2023. |