TSegNet: An Efficient and Accurate Tooth Segmentation Network on 3D Dental Model |
Zhiming Cui1, Changjian Li2,1, Nenglun Chen1, Guodong Wei1, Runnan Chen1, Yuanfeng Zhou3, Dinggang Shen4,5,6, Wenping Wang1 |
1The University of Hong Kong, 2University College London, 3Shandong University, 4ShanghaiTech University, 5Korea University, 6Shanghai United Imaging Intelligence Co., Ltd.,   |
Medical Image Analysis (MIA), 2021 |
|
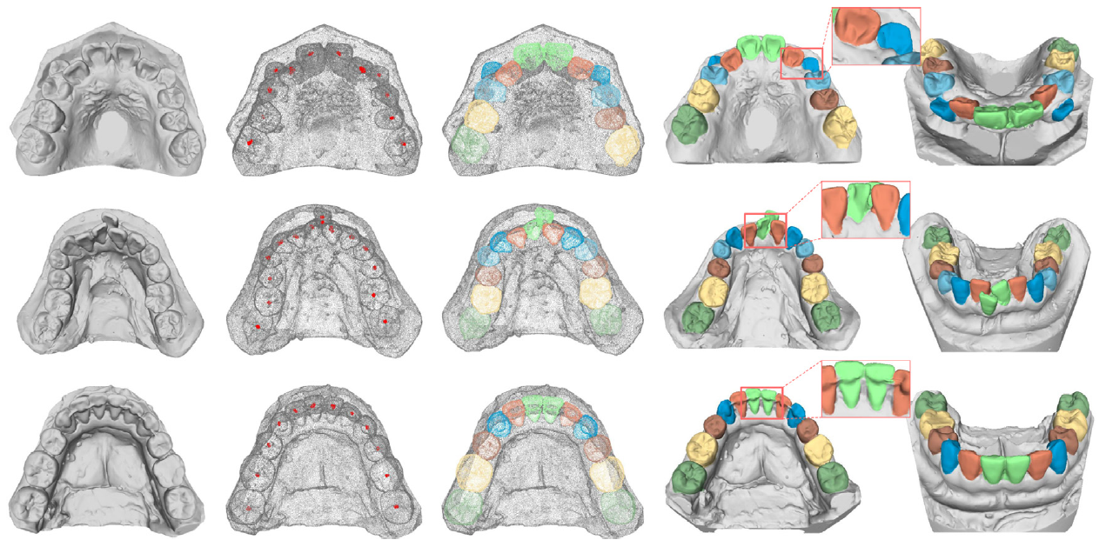 |
Fig. 1. Representative segmentation results. From left to right: input, predicted centroid points, tooth segmentation on the point cloud, tooth segmentation on dental models with two different views. The accurate segmentation boundary is highlighted in the boxes.
|
Abstract |
Automatic and accurate segmentation of dental models is a fundamental task in computer-aided dentistry. Previous methods can achieve satisfactory segmentation results on normal dental models; however, they fail to robustly handle challenging clinical cases such as dental models with missing, crowding, or misaligned teeth before orthodontic treatments. In this paper, we propose a novel end-to-end learning-based method, called TSegNet, for robust and efficient tooth segmentation on 3D scanned point cloud data of dental models. Our algorithm detects all the teeth using a distance-aware tooth centroid voting scheme in the first stage, which ensures the accurate localization of tooth objects even with irregular positions on abnormal dental models. Then, a confidence-aware cascade segmentation module in the second stage is designed to segment each individual tooth and resolve ambiguities caused by aforementioned challenging cases. We evaluated our method on a large-scale real-world dataset consisting of dental models scanned before or after orthodontic treatments. Extensive evaluations, ablation studies and comparisons demonstrate that our method can generate accurate tooth labels robustly in various challenging cases and significantly outperforms state-of-the-art approaches by 6.5% of Dice Coefficient, 3.0% of F1 score in term of accuracy, while achieving 20 times speedup of computational time. |
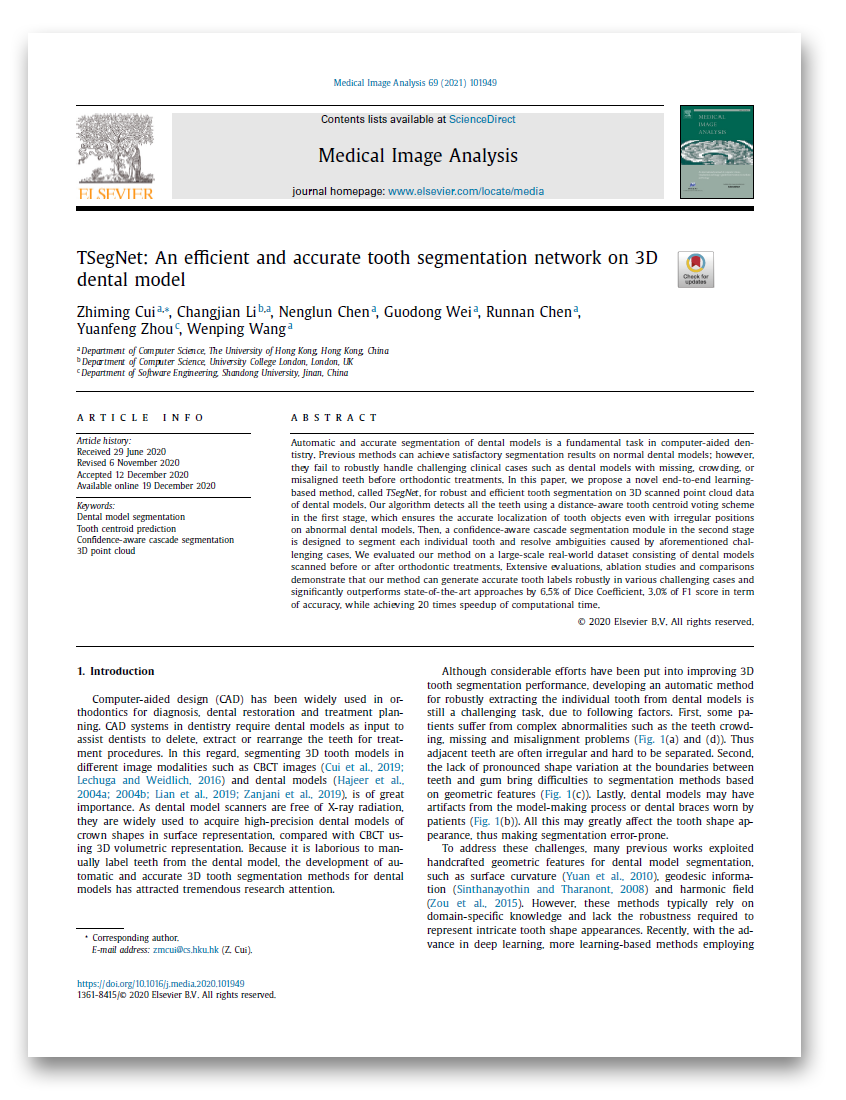 |
|
Paper [PDF]
Code [Comming Soon...]
Citation: Cui, Z., Li, C., Chen, N., Wei, G., Chen, R., Zhou, Y., & Wang, W. (2021). TSegNet: an Efficient and Accurate Tooth Segmentation Network on 3D Dental Model. Medical Image Analysis, 101949. (bibtex)
|
|
|
Network Overview |
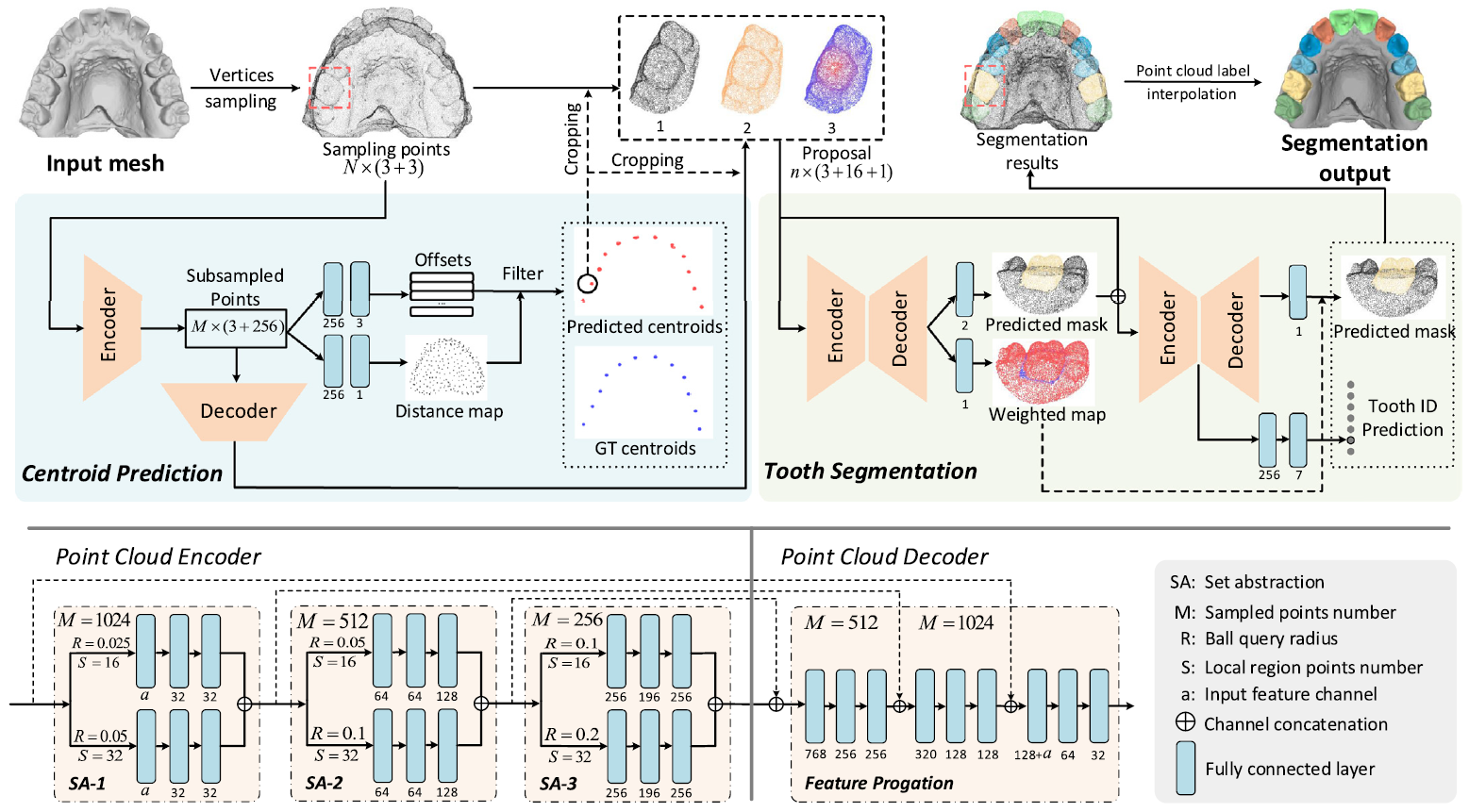 |
Fig. 2. The two-stage network architecture and the algorithm pipeline. The dental mesh is first fed into the centroid prediction network in stage one, then the cropped features based on the regressed points go through the tooth segmentation network in stage two. Finally, we derive the accurately segmented tooth objects. The numbers 1, 2, 3 in the proposal box, represent the input signals for the segmentation network, i.e., cropped coordinate feature, propagated point feature and dense distance field feature respectively. See algorithm details in Section 3.
|
|
Representative Results |
Comparison |
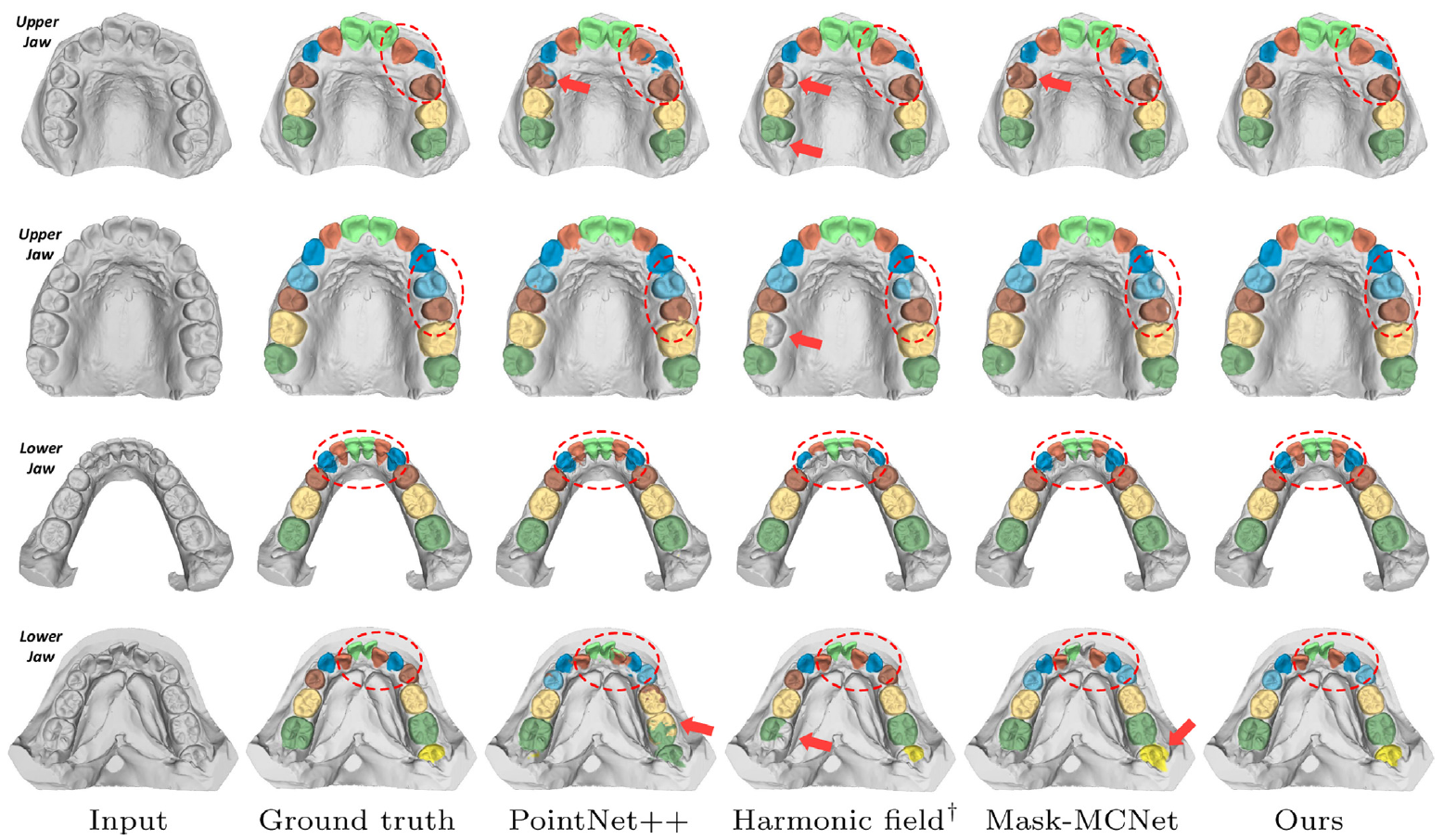 |
Fig. 3. The visual comparison of dental model segmentation results produced by different methods, with each row corresponding to a typical example of the upper or lower jaw. From left to right are the scanned dental surface, the ground truth result, results of other methods (3rd-5th columns) and result of our method (last column). Red dotted circles and arrows represent some segmentation details. ‘†’ denotes the method is a semi-automatic method. (For interpretation of the references to color in this figure legend, the reader is referred to the web version of this article.) |
Complex Cases |
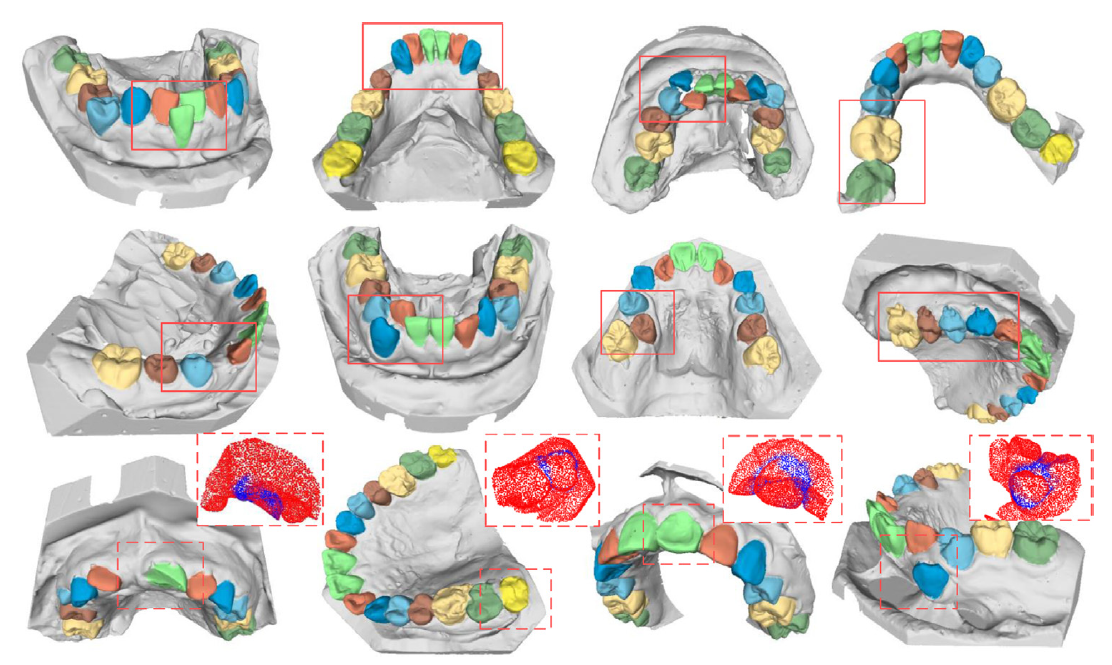 |
Fig. 4. Segmentation results of dental models with complex appearances, including teeth missing, crowding and irregular shapes highlighted by red boxes. Four attention maps of abnormal cases in the last row are also presented, and the red color indicates higher segmentation confidence while the blue color indicates lower segmentation confidence. (For interpretation of the references to color in this figure legend, the reader is referred to the web version of this article.) |
|
|
©Changjian Li. Last update: January 1, 2021. |