ToothNet: Automatic Tooth Instance Segmentation and Identification from Cone Beam CT Images |
Zhiming Cui, Changjian Li, Wenping Wang |
The University of Hong Kong |
IEEE Conference on Computer Vision and Pattern Recognition (CVPR 2019) |
|
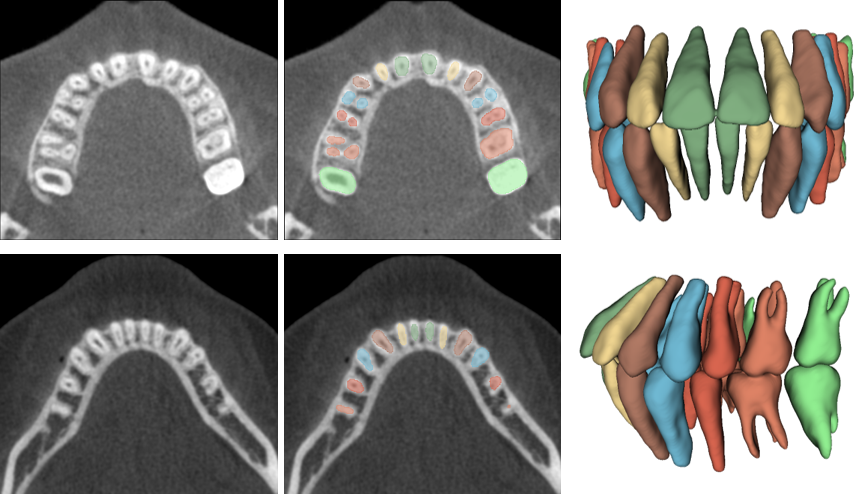 |
An example of tooth segmentation and tooth identification. The first column shows a CBCT scan in the axis view, the second column shows its segmentation results, and the last column shows the 3D segmentation results with different colors for different teeth respectively.
|
Abstract |
This paper proposes a method that uses deep convolutional neural networks to achieve automatic and accurate tooth instance segmentation and identification from CBCT (cone beam CT) images for digital dentistry. The core of our method is a two-stage network. In the first stage, an edge map is extracted from the input CBCT image to enhance image contrast along shape boundaries. Then this edge map and the input images are passed to the second stage. In the second stage, we build our network upon the 3D region proposal network (RPN) with a novel learnedsimilarity matrix to help efficiently remove redundant proposals, speed up training and save GPU memory. To resolve the ambiguity in the identification task, we encode teeth spatial relationships as an additional feature input in the identification task, which helps to remarkably improve the identification accuracy. Our evaluation, comparison and comprehensive ablation studies demonstrate that our method produces accurate instance segmentation and identification results automatically and outperforms the state-of-the-art approaches. To the best of our knowledge, our method is the first to use neural networks to achieve automatic tooth segmentation and identification from CBCT images. |
|
|
Network Overview |
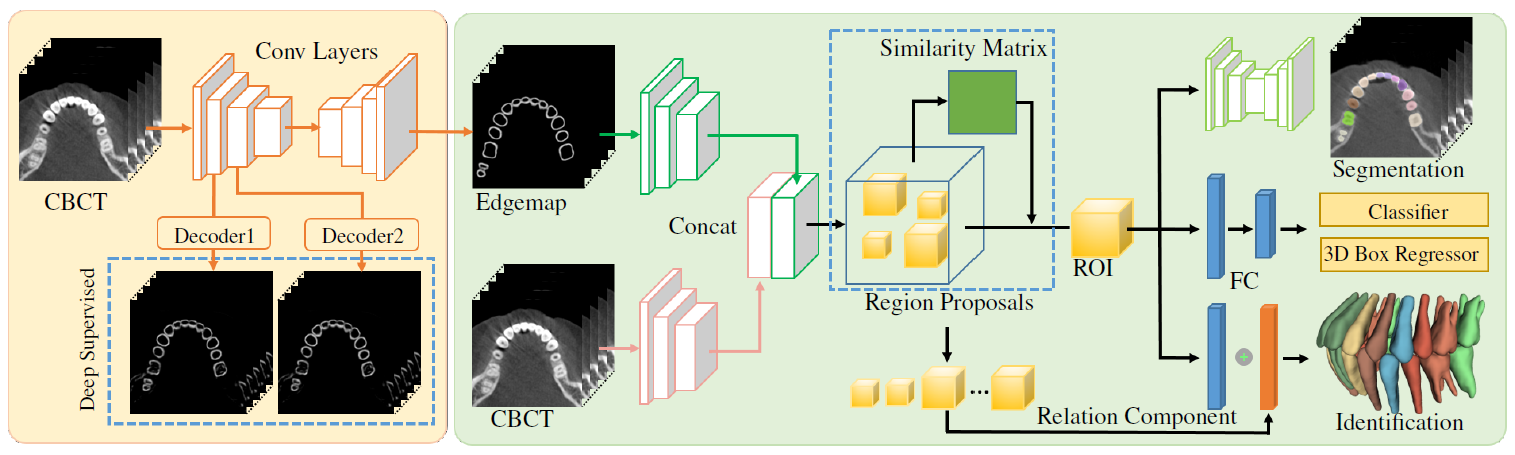 |
Our two-stage network architecture for tooth instance segmentation and identification. Given CBCT images, we first pass them
to the edge map extraction network in stage one, where the deep supervised scheme is used. Then the detected edge map and the original
CBCT images are sent to the region proposal network with a novel similarity matrix. Four individual branches are followed for tooth
segmentation, classification, 3D box regressor and identification. In the identification branch, we further add the spatial relation component
to help resolve the ambiguity.
|
|
Representative Results |
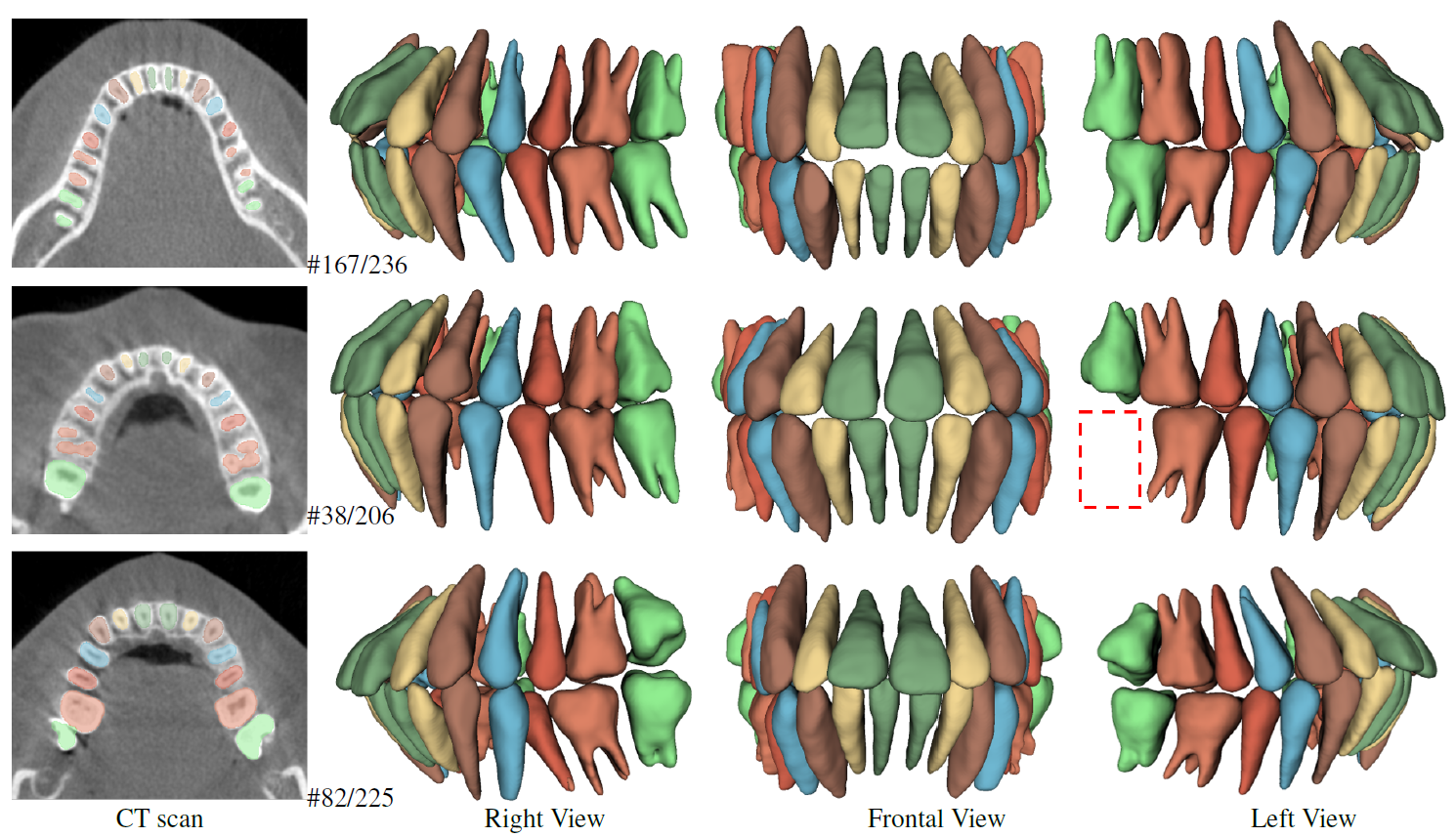 |
The results gallery of the tooth segmentation and identification. Different CT scans with segmentation results are shown in the first column, and the reconstructed 3D teeth models from three different views are shown in the following three columns. The numbers illustrate the scan indices and different colors illustrate different teeth. In addition, the second example contains a removed molar tooth, whose position is marked by the red dashed box. |
|
|
©Changjian Li. Last update: July 18, 2019. |